
Computer Vision Services for Hire
Prototyping and algorithms
Whiteboard brainstorming sessions turn to working prototypes on our day-to-day. Python or Matlab, scientific ideas can be quickly tested and expanded to new products, ready for testing and experimentation.
Coding
Algorithm development is our passion. The ingredients consist of scientific knowledge, data, requirements, and pre-developed code libraries. The process brings out creativity from everyone involved, and the result is always inspiring.
Consulting
Projects in their early steps, as well as those in need for a steering committee, are welcome to contact Vision Elements for consultancy. Early advice is worth a lot for it can change the course of a project and prevent costly mistakes.
Pre-developed code libraries
We live in a world that makes it so easy to avoid re-inventing the wheel. Open source code is our bread and butter. When that is not enough, we often have the pleasure to offer our own pre-developed code libraries to save time and money. Implementing academic papers is also part of our routine.
Product strategy
Our broad perspective on technologies lying at the core of advanced products makes us a valued partner to building product roadmaps and strategy. Young as well as mature teams that are crafting products based on remote sensing and deep algorithms may leverage the expertise gained over decades.
CTO-ship
Technological leadership is what we do for a living, and it has been our pleasure to accompany entrepreneurs in their path to growth. Staying on top of the state of the art, leading innovation, participating in funding campaigns, and drafting intellectual property applications go hand in hand with our activities.
Literature review
Conducting professional surveys to get up to speed with the state-of-the-art in domains we research, covers categorizing literature based on magazine impact factor, number of citations, relevance, reported accuracy, size of database needed, performance, and level of user intervention.
​
Patent disclosures
Proof-of-concept projects often relate to the core technology of companies. Working with patent attorneys on drafting these concepts into protectable descriptions is part of our process. Our services hence include the authoring of white papers, specifications and engineering reports.
Due diligence
Investors and acquirers who need to examine technologies at different phases of maturity in the space of AI and computer vision can benefit from the fact that our teams see dozens of companies from different domains. We have a good sense of what’s feasible and what’s working in the subject matter we cover.
Researchers for Hire
Data Science
Research
Signal Processing
SW & Algorithms
Robotics
Clinical Science
Physics & Simulation
Mapping & Navigation
Metaverse
Fusion & Multi Sensing
Computer Vision
AR & VR
Plethora of platforms
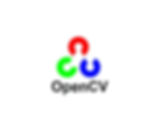

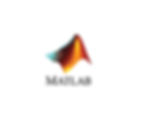
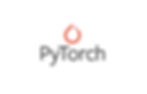
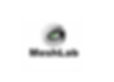

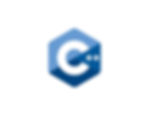





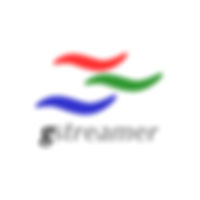
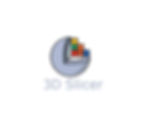






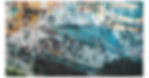
Tailor-made to maximize flexibility
Our solutions are vendor-agnostic. From the optics of the acquisition sensors, to the run-time deployment, our clients trust us to maximize speed to value and optimize cost.
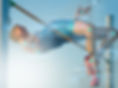
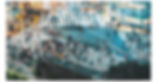
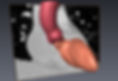
Imaging at scale
There is a huge amount of information to be extracted from different sources of imagery. Images at every scale – microscopic to astronomical – capture valuable insights from remote. Signals of various dimensions are often fused to turn even the latent of understandings to a meaning.